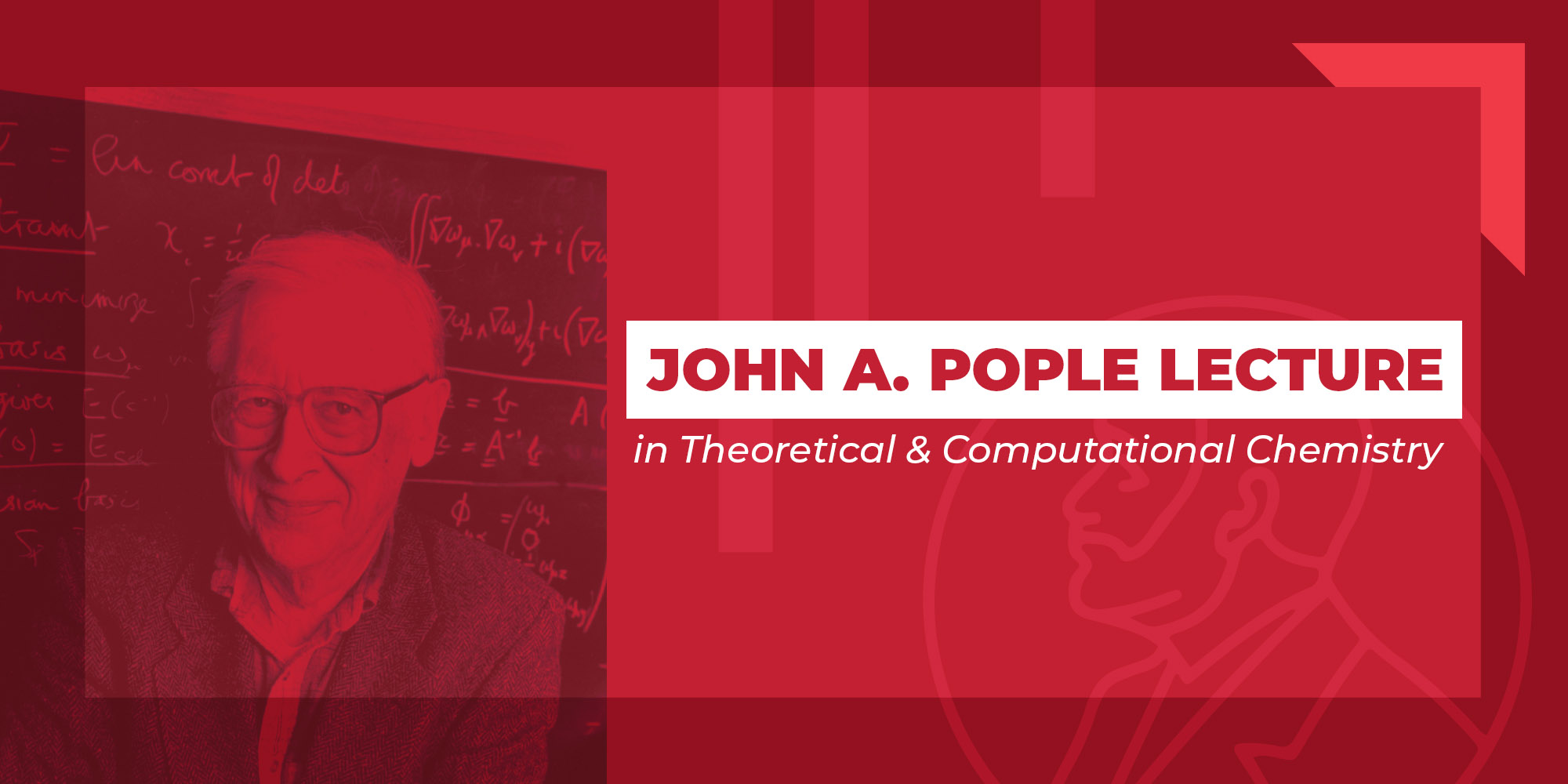
October 09, 2024 at 4:00 p.m.
Mellon Auditorium, Mellon Institute
Carnegie Mellon University
A reception will follow the lecture
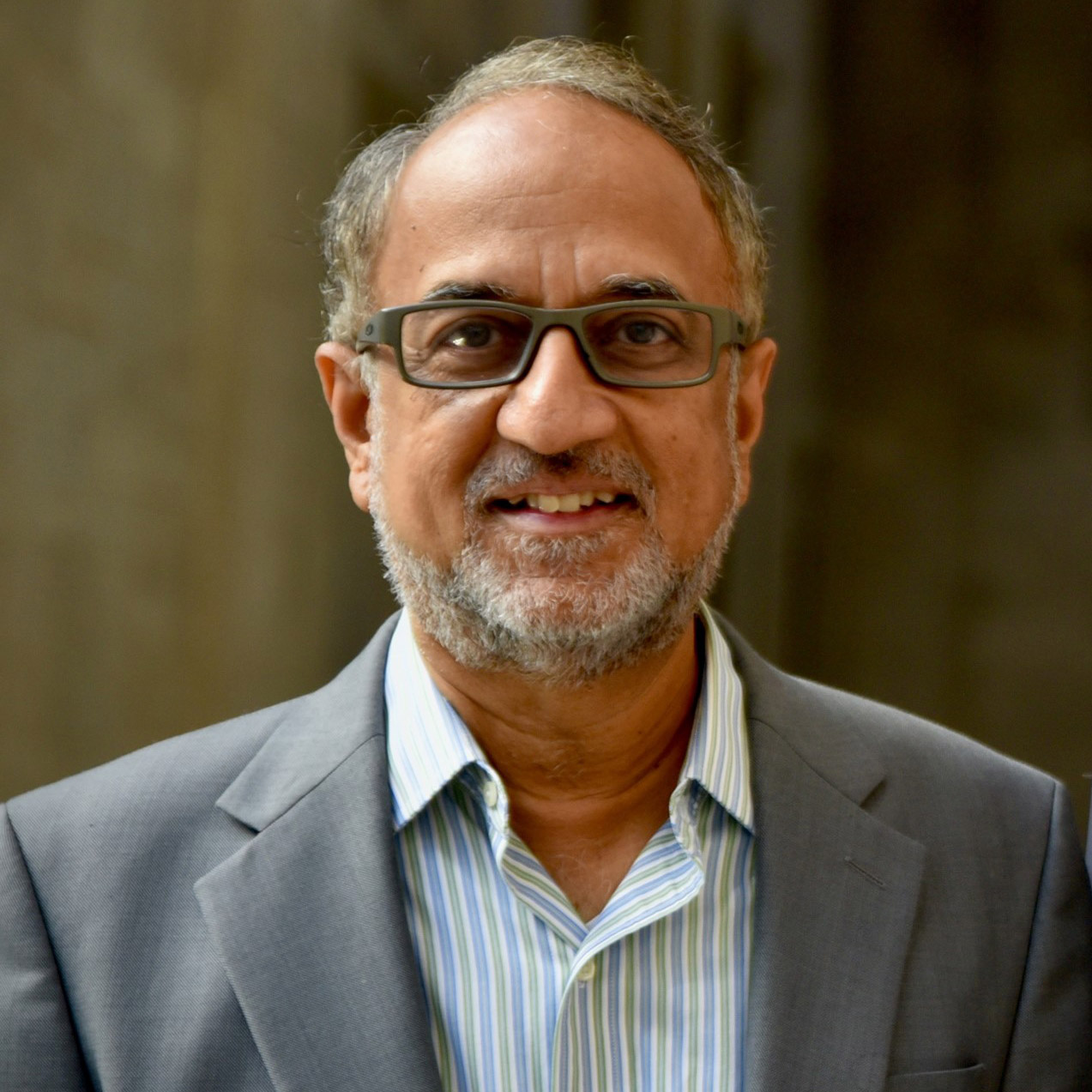
Krishnan Raghavachari
Distinguished Professor Indiana University
Achieving Coupled Cluster Accuracy at DFT Cost: Novel Ideas from Molecular Fragmentation, Error Cancellation and Machine Learning
Originally from India, Prof. Krishnan Raghavachari received his B.Sc. in Chemistry from Madras University (1973) and his M.Sc. in Chemistry from Indian Institute of Technology, Madras (1975). He received his Ph.D. from Carnegie Mellon University in 1981 working with legendary Professor John Pople (Nobel Laureate in Chemistry, 1998). He joined Bell Laboratories as a research scientist in 1981 and later received the Distinguished Researcher Award in 1987. He joined Indiana University as a Professor of Chemistry in 2002 and was appointed as a Distinguished Professor in 2014.
Professor Raghavachari is well known for his work on the development of electron correlation theories and is the principal author of the highly successful CCSD(T) technique (1989), often called “the Gold Standard of Quantum Chemistry”. Research in his group focuses on the development of accurate and insightful computational models in quantum chemistry and for pioneering their use in forefront applications ranging from semiconductor surface chemistry to computer-aided drug design. He is a pioneer in the development of fragmentation-based methods in quantum chemistry that enable quantitatively accurate studies on large molecules. He has expanded his research profile in recent years to include machine learning in quantum chemistry, and computer-aided design of fluorescent materials and receptors. He has co-authored more than 450 publications in the fields of chemistry, physics, biochemistry, and materials science, and his papers have been cited over 80,000 times (h-index=98).
Prof. Raghavachari is an elected member of the “International Academy of Quantum Molecular Science”, and is a Fellow of the American Physical Society, the Royal Society of Chemistry, and the American Association for the Advancement of Science. He has won many prestigious awards including the “Davisson-Germer Prize in Surface Physics” sponsored by the American Physical Society (2009), “Distinguished Alumnus” award at Indian Institute of Technology, Madras, (2014), Chemical Research Society of India Medal (2017), the Bicentennial Medal for Distinguished and Distinctive Service, Indiana University (2020), and most recently the American Chemical Society Award for “Computers in Chemical and Pharmaceutical Research”.
Abstract
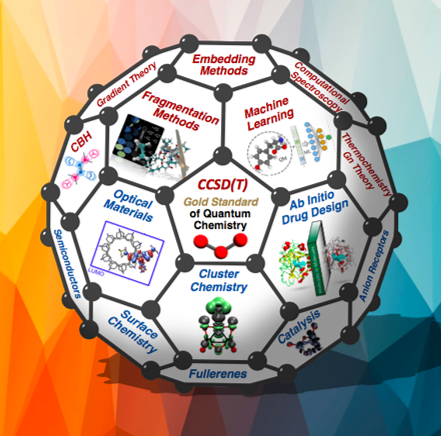